What you need to know about advertising for ecommerce in a privacy-first world
In recent years, advertising platforms have allowed marketers to become obsessed with performance—perhaps to a fault.
Where channels like Facebook and Google have made it so easy for merchants to play in the figurative sandbox, many have strayed from creation and innovation and instead become hyper-fixated on optimization, tweaking, and “growth hacking.”
But now, data privacy changes are taking marketers back to their advertising roots.
Today, there’s a renewed focus on ensuring each ad goes further, and broader—so that it’s relevant to anyone who might come across it. And it’s becoming increasingly important to zoom out from understanding and identifying niche, targeted audiences, to instead putting the brand, the story, and the product first.
But all of that is easier said than done. We talked to a few experts, analyzed a few brands, and scrolled through our social media feeds to get a better understanding of what’s working and what isn’t across the ecommerce advertising landscape.
Table of contents
- What is ecommerce advertising
- The state of advertising on Facebook and Instagram
- The paid social up-and-comer: TikTok advertising
- Other paid social channels
- The lowdown on Google Advertising
- Balancing owned marketing and paid advertising
What is ecommerce advertising?
Ecommerce advertising is a method of generating traffic, clicks, sales, and brand awareness through paid placements using third-party platforms like Facebook, Instagram, Google, TikTok, Snapchat, and Amazon. Ecommerce ads are used for targeting new prospects or retargeting those who have shown interest in attracting new customers.
But advertising in 2023 looks much different than it did just a few years ago. Between the Apple iOS 14.5 update and Google’s upcoming phase-out of third-party cookies, advertising on third-party platforms is getting tricky as marketers lose their ability to deliver highly personalized and targeted messages to prospects and have less visibility into single-channel attribution.
“It was literally like a plug was pulled. Almost overnight, we lost sight of 50% of our metrics, which was detrimental, and it changed the way that we needed to interact with these platforms as well as how we use them to find new customers,” says Dara Denney, director of performance creative at Thesis Testing.
It was literally like a plug was pulled. Almost overnight, we lost sight of 50% of our metrics.
Dara Denney, director of performance creative at Thesis Testing
“Now that we’ve lost so much of the analytics inside the platforms, we’re going after a much broader customer. In some cases, these customers aren’t as valuable, which is why people are exploring other acquisition methods—they’ve had to reduce their spend on Facebook since it’s just not as effective.”
For example, cosmetics brand Jones Road Beauty cut their spending on Facebook and Instagram by 25% while athleisure brand Feat has cut their budget on these channels by 20%, according to The Wall Street Journal.
Still, most ecommerce marketers agree that advertising continues to play a critical role in their brand’s ability to acquire new customers, which is why it’s more important than ever to understand these channels and their changes.
But in order to understand the strategies that are most effective in ecommerce advertising, you first need to have a deep understanding of the different online channels and platforms available—namely paid social and paid search.
The state of advertising on Facebook and Instagram, the paid social behemoths
Love him or hate him, it’s indisputable that Mark Zuckerberg has made a massive impact on the ecommerce industry.
In fact, some marketers insist the success of many early-stage DTC companies, ecommerce businesses, and the industry at large has hinged on Facebook’s advertising capabilities.
“As much as we love to hate on Facebook and Instagram, they deserve credit for creating this industry. Facebook Ads Manager gave merchants following the DTC business model a real shot,” says Nik Sharma, head of Sharma Brands and HOOX.
As much as we love to hate on Facebook and Instagram, they deserve credit for creating this industry.
Nik Sharma, head of Sharma Brands and Hoox
“Everybody talks about how they want to get off of Facebook, but it’s one of the largest drivers of customer acquisition for a majority of the brands out there.”
This makes sense considering Facebook and Instagram are still two of the most popular social media platforms, with 2,910M and 1,478M monthly active users, respectively, according to Statista.

But in order to make an ecommerce advertising strategy pay off on these platforms and see click-through rates soar, marketers need to adjust their thinking.
“Advertising on Facebook used to be very tactical, and it relied on Facebook’s ability to determine what creative was going to convert each person the best, based on where they were in their journey. Now, it’s all about creating a narrative that puts people on a journey, and pulls them down a conversion path,” Sharma explains.
Now, it’s all about creating a narrative that puts people on a journey, and pulls them down a conversion path.
Nik Sharma, head of Sharma Brands and Hoox
As it stands, the most important aspect of a Facebook or Instagram ad is the creative—it needs to speak to a wide range of people, rather than defined audiences or segments.
“Every piece of creative has to speak to somebody as if they don’t know the brand at all, but it also has to speak to somebody as if they know the brand completely, and they need further convincing to purchase. It’s changed the creative, and it’s really changed how we educate people on why they should buy,” Sharma points out.
“Brands really have to shift their thinking to, ‘How do we tell the brand story in a way that actually creates a fear of missing out, and influence that person to come back to us,’ versus trying to hit them at the right time and place.”
8 examples of exceptional Facebook and Instagram advertising strategies for ecommerce
So what does a successful Facebook or Instagram ad look like today, and what marketing strategies are succeeding for brands?
Here are 8 examples of brands that are creating ads to engage potential customers and existing customers alike.
1. Cadence uses storytelling to sell their “why”
With this Facebook video ad, Cadence accomplishes a number of different things through vivid brand storytelling.
First, viewers meet the founder, Steph Hon, and achieve an immediate understanding of why she created the travel capsules in the first place—to help her maintain a routine while traveling.
As the video continues, it also establishes the benefits of Cadence and signals why it’s a better option than alternatives—it’s leakproof, sustainable, magnetic, and domestically manufactured.
This particular social media ad format also highlights social proof—Cadence sold out quickly after it first launched, and it’s been mentioned in major publications such as BuzzFeed, Vogue, and goop.
“Cadence is one of the best examples of a brand that’s doing Facebook advertising right as evidenced by the fact that they weren’t very affected by the iOS change. That’s because, from day one, all of the ads that we made were focused around communicating what the product is, why it helps consumers, and what they get out of it,” says Sharma, who consults Cadence.
“They were always focused on the ‘why’—how do we make it the customer’s idea to see that this product is so great and that they need it in their life? They always focused on, ‘This is a problem our founder had, and this is what we made to solve it. We think it can help you, too.’”
2. Kinfield promotes value through earned media coverage

Instead of using your ecommerce ads to tell shoppers why your product is better than your competitors’, why don’t you let someone else do it for you?
That’s exactly Kinfield’s ecommerce marketing strategy in this Instagram advertisement that features a screenshot of the brand’s press coverage in The Strategist, titled “This Bug Spray Smells So Good My Husband (Occasionally) Wears It As Cologne.”
Kinfield’s entire mission is to make clean, effective products for people’s outdoor adventures. Their hero product, a bug spray, provides a healthier solution to other formulas filled with DEET—and anyone who’s ever used store-bought bug spray knows exactly the associated smell.
This ad simply but effectively conveys that the brand is taking a new and more natural approach to a familiar product, and hits on a pain point that many outdoor enthusiasts know all too well.
3. Omsom uses UGC to highlight high-quality products
Creating an ad with user-generated content (UGC) produced by customers, influencers, or creators is a powerful way to communicate the benefits of a product from the consumer’s point of view.
This Instagram video ad by Omsom provides specific use cases that customers might use the product for through expertly crafted UGC.
In this case, the speaker talks about how she uses Omsom starters for family dinners, holiday celebrations, and quick meals, then actually shows how she makes a recipe using the product.
This helps showcase an example of how the product is used in real life, so consumers can envision using it to create their own home-cooked meals.
4. Jones Road Beauty shows the product in action
Makeup mogul Bobbie Brown’s latest endeavor, Jones Road Beauty, is all about helping people achieve an all natural no-makeup makeup look, while simplifying their routine to just a few products.
This Facebook ad features Bobbie Brown herself, already establishing the brand with a familiar face that people trust. The rest of the video takes an educational approach, helping shoppers decide which miracle balm is right for them.
She explains the difference between all the colors and shows the product in action to better demonstrate what the application looks like. If shoppers still have questions, Brown directs them to the onsite Octane AI quiz, where they can answer a number of questions to get their personalized color match result.
5. ZitSticka promotes an offer people can’t refuse

ZitSticka keeps it simple yet effective with this Facebook ad, which promotes the product, its benefits, and an exclusive offer for social media scrollers.
For people that haven’t heard of the brand, or for those who have, this ad serves as an enticing way to get people to try the skincare brand’s namesake pimple patches, all the while incentivizing a higher average order value on each purchase.
ZitSticka shows you don’t always have to have a highly produced video ad to stop the scroll—sometimes all it takes is vibrant imagery, clear and concise language, and a compelling incentive to get people to take notice.
6. House of Wise promotes a solution to a common problem through content

Rather than promoting their sleep CBD gummies, House of Wise uses their image ads to promote an article which lives on their blog titled, “How to Use CBD to Improve Sleep Quality.”
For people who may be on the fence about CBD and its benefits, this article bridges the gap between the awareness and consideration stages in the buyer’s journey to more effectively convince shoppers that CBD belongs in their daily routines.
By piquing people’s curiosity, House of Wise effectively convinces shoppers of the advantages of CBD and familiarizes them with the brand all in one fell swoop.
7. Each and Every evokes urgency
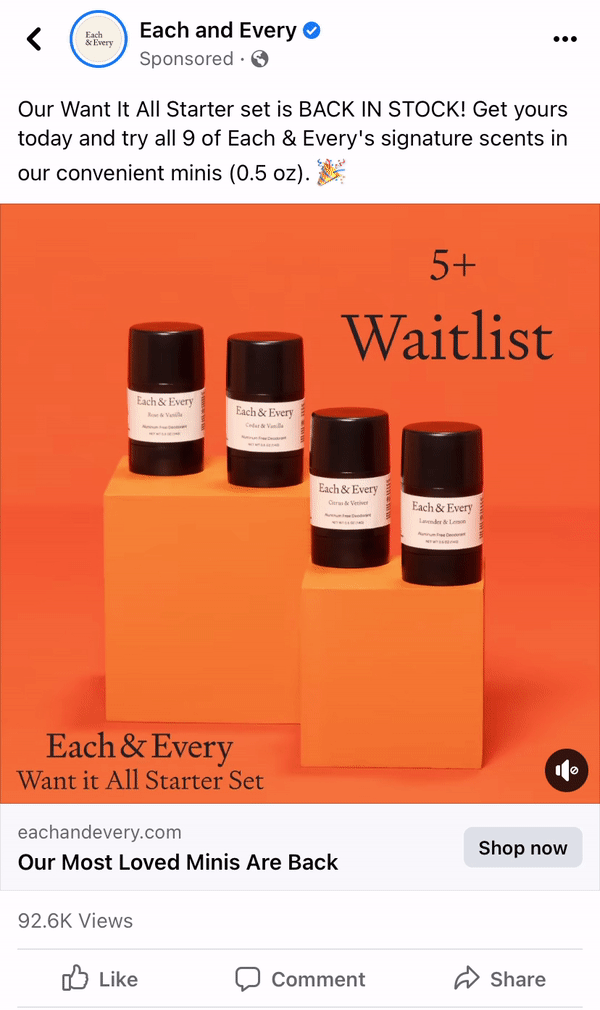
This simple GIF by Each and Every establishes a few important points in the brand’s favor—the fact that their hero product sold out, had a 1K-person waitlist, and is finally back in stock.
The GIF evokes a fear of missing out among consumers who didn’t have a chance to snag the brand’s starter set the first time around, while also creating a sense of urgency—buy now, before we sell out again.
Whether you’ve been to Each and Every’s ecommerce website once or a hundred times, this ad appeals to shoppers who don’t want to miss out on the highly sought-after minis.
8. Brightland highlights a best seller
Even though Brightland has a variety of flavored olive oils, they use this Instagram video ad as an opportunity to specifically focus on Rosette, their garlic olive oil.
The ad features reviews and testimonials that speak to how customers use the oil to complement different dishes, and showcases the recipes they refer to in the background.
This is a sensory way to leverage social proof and make it even more mouth-watering for hungry shoppers.
The paid social up-and-comer: TikTok advertising and the brands that are doing it right
Facebook and Instagram pioneered the paid advertising front and other platforms haven’t even come close to their combined level of popularity.
Even though TikTok still doesn’t quite compare to Meta’s mega presence, the platform is certainly gaining traction, specifically among Gen Z and millennial consumers—and marketers are taking notice.
“What makes TikTok such a good opportunity for ecommerce marketers right now is that the user journey and the way that people interact with the platform is completely different than Facebook or Instagram—people are using it as an entertainment platform, similar to Netflix,” says Denney.
People are using [TikTok] as an entertainment platform, similar to Netflix.
Dara Denney, director of performance creative at Thesis Testing
TikTok currently has 1.2B monthly active users, officially beating out social media marketing platforms including Snapchat, Pinterest, and Twitter—and just last year, it was the most downloaded app worldwide. Additionally, 70% of TikTok users spend an hour or more on the app weekly, according to Statista.
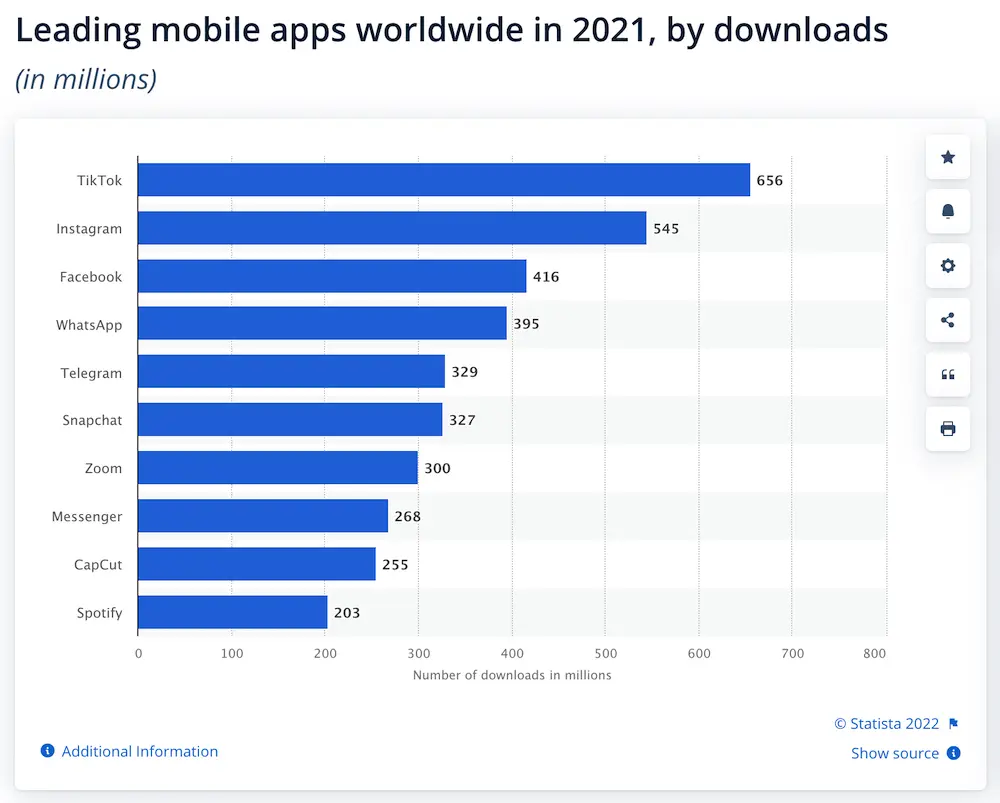
The numbers don’t lie, but TikTok also has a certain je ne sais quoi—the music is catchy, the trends are compelling, the content is quick-hitting, and the creators are insanely talented.
If marketers can capture the essence of TikTok in their ad creative and manage to entertain to a similar degree as these creators, they’re almost certain to elicit engagement—not only on TikTok’s native platform, but across other social media platforms as well.
“When we partner with creators to develop trendy content that has the same pacing and text treatments as TikTok or that uses popular sounds, we find that those do great on TikTok, but they also do really good on Facebook and Instagram, so we’re starting to double down on that,” says Denney.
For example, Denney has found that leaning into TikTok’s native treatments, like response bubbles, has served as an engaging hook for ads across paid platforms.
“This is great area to split-test. If we want to try that strategy out with a client, we’ll ask 4-5 different questions in these TikTok response bubbles, and it’s interesting to see what people are attracted to,” Denney explains.
“Sometimes we’ll ask value-based questions, sometimes we’ll ask a more FAQ-style, other times we’ll be completely nonsensical—and sometimes it’s the nonsensical, clickbait questions that get the good conversion rate.”
Best practices & tips for ecommerce advertising on TikTok
The key to understanding what will work best on TikTok is to spend time on the platform and pick up on the patterns you see—or even check out the TikTok top ads library.
Other ways to make your content algorithm-friendly and incorporate TikTok into your ads may include:
- Using popular TikTok music. When you scroll through TikTok, you’ll typically notice that certain songs are repeatedly used across almost every video. Try using these trending songs in your ads.
- Incorporating TikTok creator challenges, themes, and running jokes. Take notice of the trends you’re seeing creators follow on TikTok, and try to mirror or recreate them with your own ad creative.
- Leveraging TikTok soundbites. TikTok creators often repurpose the same soundbites and overlay them onto different situations to change the meaning. You can use these soundbites in your ads and relate them back to your brand or product.
For example, this TikTok ad from Brooklinen references “No Bones Day,” a TikTok trend made viral by Noodle the Pug, and features the “No Bones Day” song a fellow TikToker made in Noodle’s honor.
By integrating these elements in their ad, Brooklinen manages to include relevant TikTok trends while also evoking a sense of familiarity and excitement among any passive scroller who may be familiar with Noodle.
Meanwhile, this ad from Curie Bod actually lives on Instagram but incorporates many of the styles and treatments you would find on TikTok. It effectively tells the story of the brand through the founder, Sarah Moret, in a way that’s entertaining, informative, and authentic—not to mention, who can resist a Shark-backed brand?
When it comes to TikTok advertising, and creating ads inspired by TikTok videos, the less you can make an ad feel like an ad, and the more you can make it feel like a form of entertainment, the better.
“You have to remember that people aren’t on social media to look at ads. They’re not there to buy stuff. They’re not there to get to be tricked into clicking on something. They’re there to be entertained, and if you can fit into that, you’ll succeed. That’s why everybody’s favorite marketing campaign is some form of entertainment to them. And that’s why people go crazy for Super Bowl ads—they’re off-the-wall entertainment,” says Sharma.
You have to remember that people aren’t on social media to look at ads. They’re not there to buy stuff. They’re not there to get to be tricked into clicking on something. They’re there to be entertained.
Nik Sharma, head of Sharma Brands and Hoox
“Rethink your ad strategy and ask yourself, ‘Is this something that’s entertaining to somebody who’s scrolling on social media?’ If it’s not entertaining, it might work, but probably not as well as if it was.”
Other paid social channels, and how to decide which is right for your brand
In addition to Facebook, Instagram, and TikTok, brands advertise on a number of other social media advertising channels, including:
- Snapchat
Many marketers have started experimenting with paid ecommerce advertising on these channels as a way to diversify their ad spend and move away from Facebook and Instagram post-iOS 14.5.
So how do you know what paid social channel is worth your time and money?
Nick Shackelford, CEO and managing partner at Structured Agency, emphasizes that, above all else, marketers should consider the daily active users and demographics of each channel, and understand how that maps to their target customers.
“We looked at TikTok in Q1 of 2022, and their daily active users were increasing in the 17-38 age range, which is our target demographic, so that’s the hottest play for brands like ours right now,” says Shackelford.
“Some people are on Snapchat. Some are on Instagram. If you look at YouTube, the daily users are broad and consistently growing. Another brand might want to create an ecommerce advertising strategy to speak to a specific demographic there, and they could do really well because there are both kids and parents spending time there.”
Additionally, it’s important to compare product margins to the cost per click (CPC) and cost per impression (CPM) of each platform when thinking about which marketing channels will provide the highest returns for your business.
“If you have a product that sells for under $40, it’s really difficult to get working, especially on Facebook and Instagram where you have CPCs and CPMs in the $10-$20 range. Whereas on other social media platforms, you probably have a little bit cheaper cost of traffic,” says Shackelford.
No matter what, you should always be testing with your online advertising strategy, and adjusting your creative or the channels you’re deciding to spend on if it’s not working for your business.
“Test—a lot. Every advertisement that you launch should be leveraging A/B testing in one capacity or another,” says Spencer Flaherty, internal marketing manager at Groove Commerce.
“While modifying your copy and images is a great way to test their individual performance, be sure to only change one element at a time in order to correctly attribute success or failure,” he adds.
Additionally, Sharma advances that your ecommerce advertising strategy should be a balancing act between sticking to proven tactics and exploring new ideas.
“I’m all about 80/20—80% go with what works, 20% experiment and try new things,” says Sharma.
The lowdown on Google advertising for ecommerce brands
Google is by far the most popular search engine, taking up more than 85% of the market share of search engines and far outperforming competitors like Yahoo and Bing.
Google also processes over 40K search queries per second and fields more than 3.5B searches per day
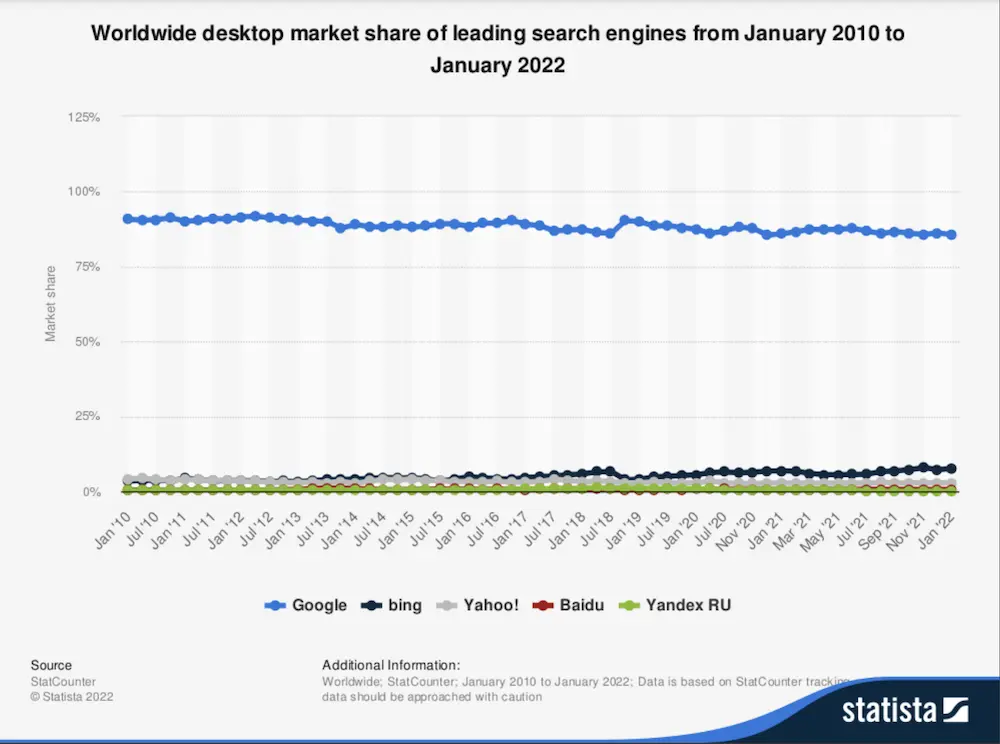
This makes Google and its ad platform, Google Ads, an extremely attractive place to advertise an ecommerce brand.
There are a few ways to advertise your brand through Google:
- Search (text ads on Google search results)
- Display (banner ads on websites)
- Shopping (product listings on Google)
- Video (YouTube)
- App (GooglePlay)
But data privacy changes are creating turbulence with Google advertising, as well.
What’s happening with third-party cookies and banner ads
The third-party cookie conundrum is very similar to what’s happening with iOS 14.5—in the interest of consumer privacy, Google decided that third-party cookies are too invasive.
Third-party cookies are the little pixels that track peoples’ activity across the internet. Advertisers use these cookies to target consumers with ad campaigns that are relevant to their browsing history.
The biggest threat of disappearing third-party cookies is to display advertising, or the highly targeted ads you see across different websites. Although display ads will still work, the targeting will likely be less effective.
That being said, display ads can still be very effective for growing brand awareness. For example, if you run an active lifestyle brand that sells workout gear, you may want to place ads on fitness-related blogs and websites.
Even if targeting eventually won’t be as precise in telling you which users looked at which websites, you can use the context of the website to inform ad placement.
Google is also planning to test Federated Learning of Cohorts (FLoC) to ease the burden of disappearing third-party cookies. FLoC tracks groups of people based on their common interests or behaviors, rather than individuals.
As a result, marketers will need to learn how to target these groups of users—once again appealing to broader audiences, instead of hyper-targeted personas. And many experts think there will be an increased emphasis on strategic paid search advertising in order to get the most out of Google.
Paid search strategies that don’t depend on cookies (+5 brand examples)
Paid search refers to promoting your website through search engines like Google, Yahoo, and Bing by placing ads on users’ search results, either through text ads or Google Shopping ads.
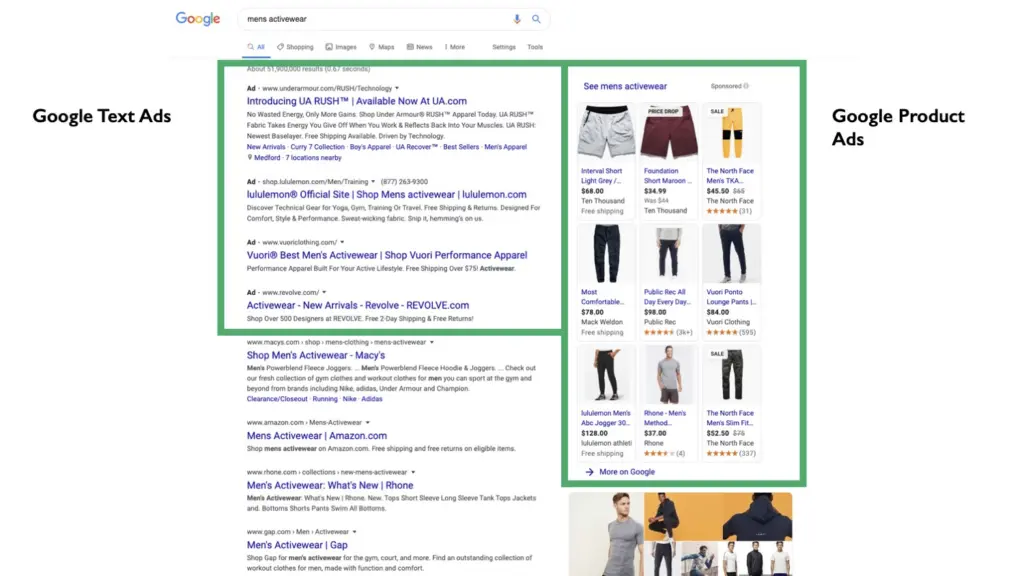
This is also known as search engine marketing (SEM) or pay-per-click (PPC), since marketers pay for these ads based on how frequently a user clicks on them.
It’s also not to be confused with search engine optimization (SEO), which refers to getting your ecommerce website to rank organically through content marketing.
But when should you use paid search advertising for your ecommerce business?
The important difference between paid search and paid social is the intent level on each channel.
When someone searches for something in Google, they have a high level of intent to find a specific product or result. Meanwhile, social media is a more passive discoverability channel—people aren’t usually seeking out a certain product.
With that in mind, Shackelford encourages marketers to consider the product they sell and the level of education around it when considering whether to try paid search advertising.
“People around the world are familiar with sunscreen, know what it is, and understand why they need it. So a sunscreen brand like Coola knows that there’s already a large intent of search around it, which makes it a perfect fit for paid search,” says Shackelford.
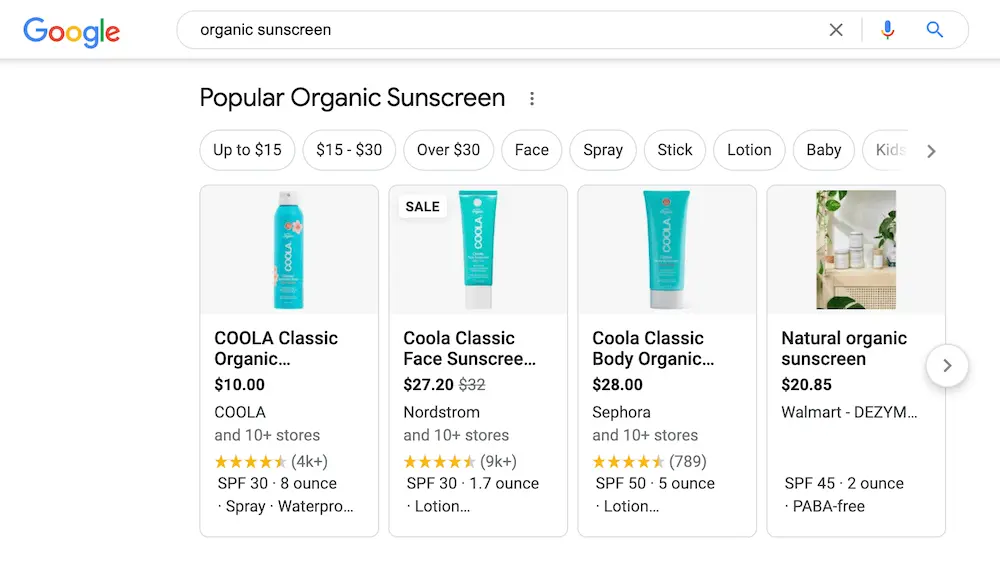
“On the other hand, you might have a company like Cometeer. People are always searching for coffee, but Cometeer is a little bit more unique because it’s sold as frozen capsules. They already know that there’s a volume of searches there for coffee, and so they can take advantage of that through paid search.”
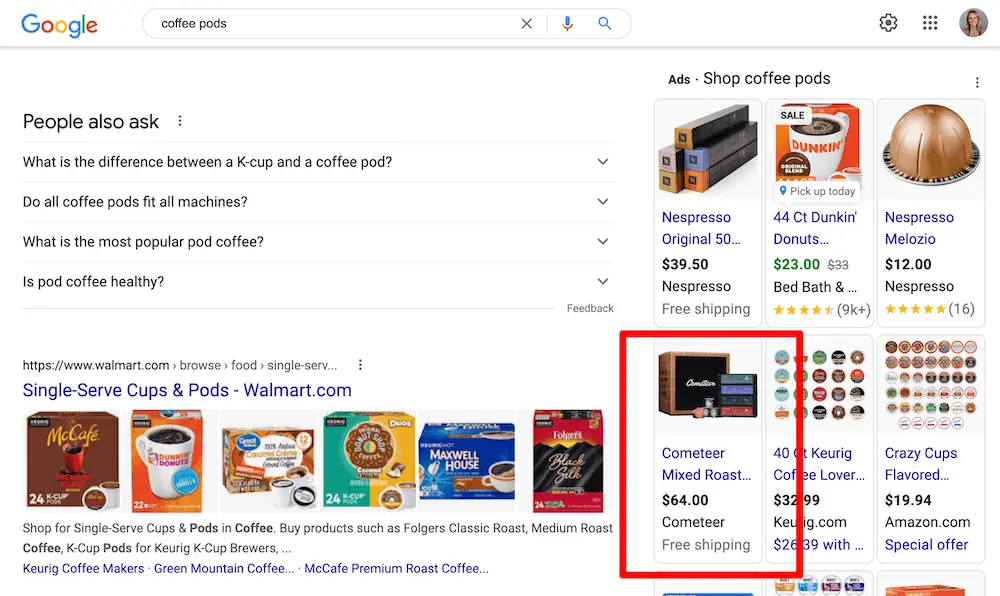
For products that are newer to the market or are more inventive and could benefit from brand awareness, paid social might be a better option than paid search because it enables you to educate the passive scroller.
But there’s another option for these types of brands, which is creating paid search content to speak to a more specific search term. A great example of this is with brands in “taboo” categories like CBD.
“Every advertising channel, including Google and Facebook, has a policy around the way you can position CBD or hemp, so you have to approach it from an education-first standpoint. The only way you can drive traffic is if the buy button is at least two clicks in, and you’re driving purchases through information and content,” Shackelford explains.
“There are times where a brand is on the fringe, you have to provide education, or there’s specific use cases that people might not come up with on their own—that’s a perfect time to have content for paid search advertising.”
For example, beam offers a variety of functional wellness products, where the main ingredient is hemp.
If someone searches for “all natural sleep aid” in Google, one of the first results is a sponsored ad from beam that leads to an article called, “Why You Should Nix Sleep Aids and Use Natural Sleep Supplements Instead.”
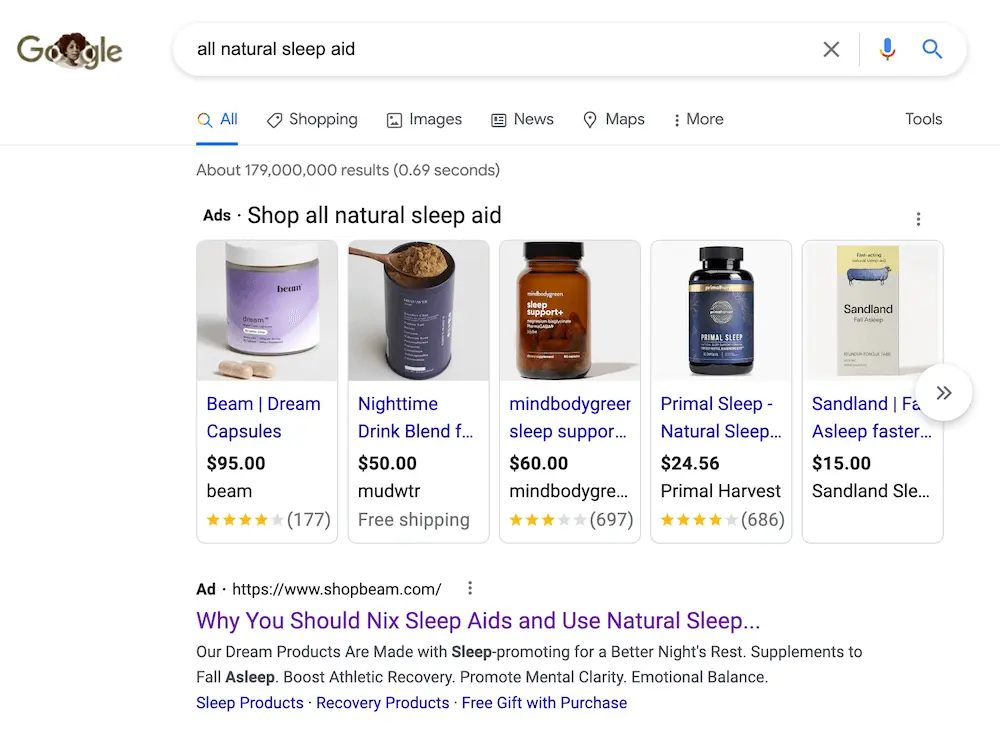
Rather than taking people who are looking for a natural sleep aid straight to beam’s product page, they take the opportunity to create education around their brand, their products, and the benefits of natural supplements. The article contains a call to action to shop for their sleep product, Dream.
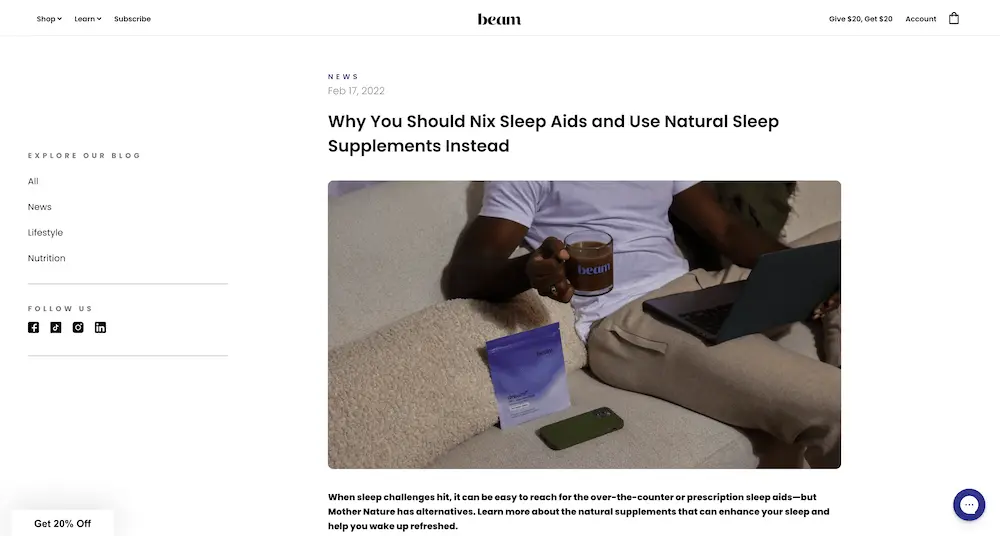
But your ecommerce site doesn’t have to exist in a “taboo” industry in order to use this method—any time you think there may be a good opportunity to provide more education around your brand or product pertaining to a search term, you might want to consider a content-based approach.
And when asked whether he thinks it’s going to be more important for brand marketing teams to invest in that type of content as part of their advertising strategy, Shackelford replied, “I completely and honestly do.”
Not every team is going to have a dedicated content marketing strategist or writer to create a corresponding article for every search advertisement, though. Even so, at a minimum, you should ensure the product or landing page you set up effectively educates shoppers about how your product meets their search criteria.
“Don’t assume people know what you do and why they should care,” suggests Loretta Doria, director of strategy at Ragnarok.
“When you’re marketing a small business, you’re usually wearing all the hats, which makes it harder to see the forest through the trees. When you think about advertising, take a step back and use a customer’s mindset as your guide for how you educate on your offering.”
Don’t assume people know what you do and why they should care.
Loretta Doria, director of strategy at Ragnarok
Lastly, marketers may want to experiment with targeting branded keywords through paid search.
One reason to do this might be that your brand name has a high search volume, but the intent of the search is often for something totally different. Paying for a branded search term ensures that the people who are seeking out your website can find it more easily.
For example, Cadence sells capsules that are ideal for traveling, but someone who’s searching for “cadence” might be looking for any number of different things. The brand ensures their website is one of the first results someone sees when searching for the term.

On the other hand, marketers might want to target competitor’s branded keywords and offer an alternative solution to a highly searched product or company.
Luggage brand Monos does just this by targeting the branded keyword “away luggage” in their paid search ads and promoting their competing products as the “Best Luggage” of 2022.
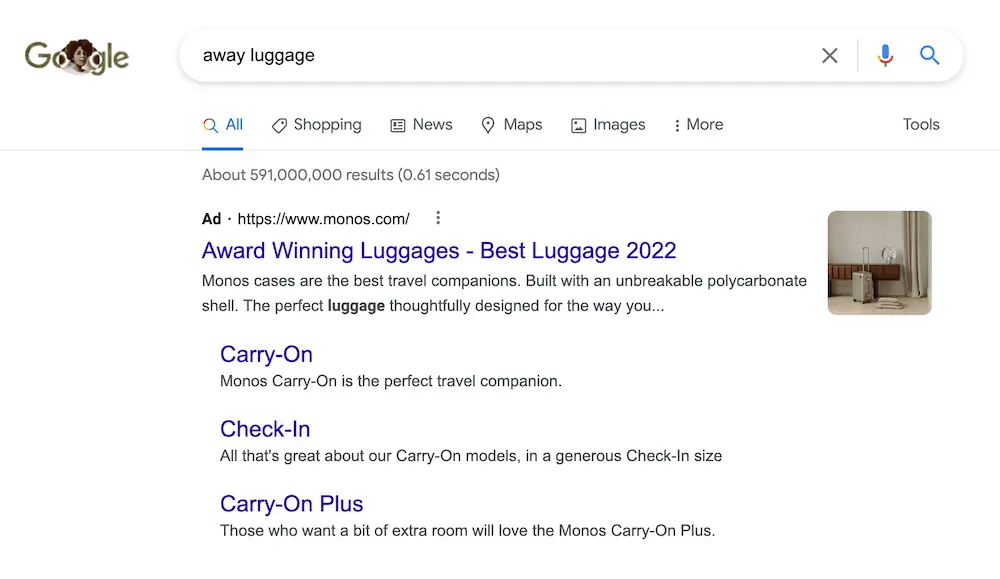
Since the term “away luggage” has a monthly search volume of 165K, according to Semrush, it makes sense for a competing brand to target this keyword in order to try to convert some of that high-intent traffic to their own brand.
An eye towards owned marketing: the importance of creating thoughtful onsite experiences
Perhaps the most important consideration with digital advertising other than the ad creative itself is what happens after someone clicks it.
“If it was my choice, every source of traffic would have a different web experience, whether someone comes from TikTok, Snapchat, email marketing campaigns, SMS, paid search, or a TV ad. Most homepages don’t really tell you anything, and the idea that a homepage should be your universal storefront doesn’t make sense,” Sharma says.
Most homepages don’t really tell you anything, and the idea that a homepage should be your universal storefront doesn’t make sense.
Nik Sharma, head of Sharma Brands and Hoox
Sharma emphasizes that landing pages are often an untapped opportunity for marketers to relay the most important information to shoppers and build a better customer experience.
“The landing page is where you get the opportunity to roll out the red carpet, walk somebody through the brand, and teach them everything they need to know based on their intent. Landing pages let you deliver information in a condensed but effective way, without making the shopper click around to seven different links, or leave the site to go look up something about the brand,” he explains.
For example, this landing page from Caraway was created to be the catch-all for paid traffic coming from Google, Facebook, and its affiliate partners.
By creating education around Caraway’s products and supporting it with social proof, Caraway reported a 74% increase in conversion rate on this page.

“The cart abandonment rate is more than 80%, which is crushing brands. For net new customers, it’s imperative you get them to convert as quickly as possible,” urges Jason Yoong, head of partnerships at Ownit.
“Build a personalized landing page that brings all purchase decision information upstream, including price, shipping, hero SKU selection, payment options, and online sales channel preference options, and combine that with a two-click checkout. We’re seeing this marketing strategy lift conversion by at least 30% from Facebook paid ads.”
Additionally, a well-rounded lifecycle marketing plan includes thinking about how to grow your SMS and email list.
Don’t forget to use landing pages as an opportunity to collect Customer-First Data™, such as shoppers’ phone numbers and email addresses, so that you can stay in touch with them and re-engage them later if they don’t decide to complete checkout. Considering 92% of shoppers don’t purchase on the first visit to an ecommerce store, that’s highly likely.
Many marketers are also using landing pages as an opportunity to go beyond the basics and collect more robust Customer-First Data through interactive content like quizzes, which serve a dual purpose—they both create an engaging user experience, and they enable marketers to better personalize emails and texts based on shopper preferences.
“The biggest untapped opportunity in ecommerce right now is using a quiz as the landing page for your ads,” says Ben Parr, co-founder and president of Octane AI.
The biggest untapped opportunity in ecommerce right now is using a quiz as the landing page for your ads.
Ben Parr, co-founder and president of Octane AI
“Sending ad traffic to your quiz is the ultimate retargeting strategy. Once you’ve captured a customer with your quiz, it’s easy to remarket to them with email or SMS automation—and generate millions in ecommerce sales in the process.”
A new era of paid advertising
As far as DTC growth tactics go, ecommerce advertising isn’t going away any time soon.
But the new era of data privacy has only just begun, and it’s going to continue to test marketers to dig a little deeper, get a little more imaginative, and think about digital marketing and advertising a little differently in order to drive sales.
Instead of doing what has always been done, marketers need to pivot, and try to appeal to as many people as possible instead of appealing to as many audience segments as possible.
Lean on creativity, keep ads general, and save the personalization for content on your owned channels.
Ecommerce advertising FAQs
What are some of the most effective ecommerce advertising techniques?
The most effective advertising strategies that have delivered outstanding results for ecommerce brands in 2023 include:
- Personalization
- Mobile-first marketing
- Omnichannel marketing
- Retargeting Ads
- CRO strategy
- Affiliate marketing
- Influencer marketing
What are the best ecommerce advertising platforms?
The best advertising platforms ecommerce businesses can leverage to reach out to their target audience include:
- Search engine marketing platforms (Google Ads, Bing Ads)
- Social media advertising platforms (Facebook Ads, Instagram Ads)
- Google Shopping
- Youtube Ads
- TikTok
What is performance advertising for ecommerce?
Performance advertising is a strategic approach to marketing designed to help ecommerce brands increase sales. It is a subset of digital marketing that represents a combination of paid and brand marketing activities for which the affiliates and marketers are paid only when the lead completes the desired action.